Wealth management is a fast-paced and customer-centric field. Adopting the latest innovations is imperative to achieving customer satisfaction while maintaining profitability. Quality engineering (QE) solutions, the backbone of digital transformations, play a pivotal role in empowering wealth management organisations. Digital solutions are user-friendly, responsive, reliable, and compliance-regulated.
From compliance to data analysis to client servicing, QE is revolutionising wealth management through Natural Language Processing (NLP) and Machine Learning (ML), the two AI subsets. In this blog, we will explore the need and role of QE in achieving digital transformation in wealth management.
Need for Digital Transformation in Wealth Management
Efficient wealth management requires skimming a million data points (perhaps even more). NLP processes vast textual data, extracting insights and sentiments, while ML algorithms learn patterns and trends.
Integrating NLP, ML, and human intervention enables a holistic understanding of market dynamics, helping financial advisors and wealth managers respond effectively to evolving trends and sentiments in real time.
According to a report by Accenture1 conducted on 500 FAs from the US and Canada, 9 out of 10 financial advisors (FA) believe AI can organically grow their business by over 20%. The attached infographic provides greater insights.
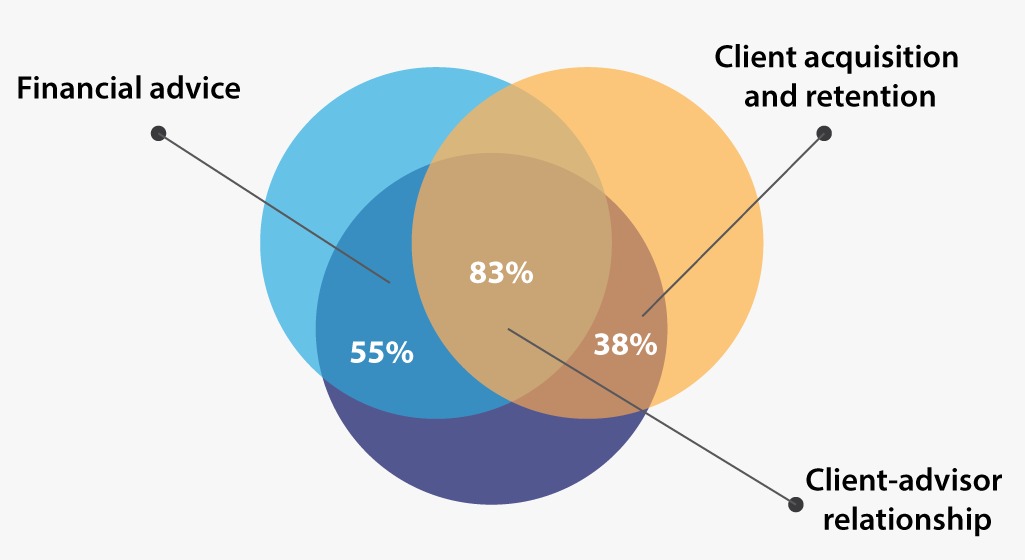
The world of generative and predictive AI in wealth management is vast. Some common AI tools reshaping high-net-worth investing are:
- Leeway Hertz’s Zbrain
- BlackRock’s Aladdin
- IBM Watson
- RavenPack
- DataRobot
Role of AI in Quality Engineering for Wealth Management
AI plays a crucial role in quality engineering for wealth management by enhancing the testing processes’ efficiency, accuracy, and scalability and ensuring the quality of financial software applications. Here are key ways AI contributes to digital transformation in wealth management:
- Automation: AI-powered testing tools and frameworks automate various aspects of testing, including functional, regression, performance, and security testing. AI algorithms can generate test cases, execute tests, analyse results, and identify anomalies faster and with higher accuracy than manual testing methods. This accelerates the testing cycle and improves software quality.
- Predictive Analysis: AI algorithms can analyse historical data and patterns to predict potential software defects or performance issues before they occur. Predictive analytics can proactively identify risk areas, prioritise testing efforts, and prevent possible failures. This reduces downtime and improves reliability.
- Data Quality Management: AI-driven data quality management tools can monitor, cleanse, and validate financial data with high accuracy and compliance with regulatory standards. This is critical for maintaining data integrity, mitigating risk, and informed decision-making in wealth management.
- Anomaly Detection: AI algorithms can detect anomalies or outliers in large data or system behaviour for potential fraud. Anomaly detection in quality engineering helps with early irregularities identification, investigates root causes, and takes corrective actions to maintain system integrity and security.
- Natural Language Processing: NLP technologies can automate the analysis of regulatory documents, compliance reports, customer communications, and legal agreements relevant to adherence to regulatory requirements.
- Chatbots and Virtual Assistants: AI-driven chatbots and virtual assistants can be used in wealth management to automate customer support, troubleshoot issues, and provide real-time feedback to users. This improves user experience, reduces manual intervention, and enhances overall service quality.
- Continuous Monitoring and Feedback: AI-enabled monitoring tools can continuously monitor software applications’ performance, security, and compliance in real-time. These tools provide actionable insights, alerts for potential issues, and feedback loops for iterative improvements, ensuring ongoing quality assurance and risk management.
Challenges in Adopting AI & ML in Wealth Management
Adopting AI and ML in wealth management poses several challenges along with numerous benefits:
- Data Quality and Availability: AI and ML models require large volumes of high-quality data to train effectively. Financial data, including historical market trends, customer transactions, and investment portfolios, must be accurate, reliable, and accessible.
- Data Privacy and Security: Financial data is highly sensitive and subject to strict regulatory requirements. Implementing AI and ML solutions in wealth management necessitates robust data encryption, access controls, and compliance with regulations such as the General Data Protection Regulation (GDPR) and the California Consumer Privacy Act (CCPA).
- Algorithmic Bias and Fairness: AI and ML algorithms can exhibit bias if trained on faulty datasets or designed with inherent biases. This could lead to unfair client treatment, inaccurate risk assessments, or skewed investment recommendations. Ensuring algorithmic fairness and transparency is crucial to building trust and avoiding ethical dilemmas.
- Interpretability and Explainability: AI and ML models, particularly complex deep learning models, can lack interpretability, making it challenging to understand how they arrive at specific decisions or predictions. Explainability is essential for compliance, risk management, and client trust in wealth management. Financial institutions must balance model accuracy with interpretability to ensure transparency and accountability.
- Regulatory Compliance: The financial industry is highly regulated, with strict compliance requirements governing investment advice, risk management, anti-money laundering (AML), and Know Your Customer (KYC) procedures. These compliance requirements involve expenses for wealth management firms. For instance, Fenergo’s report ‘Global KYC Trends in 2023’ observed that the average cost of carrying out a single KYC increased by 17% since 2022. Quality engineering like Qualitest can help reduce these costs by customisation.
- Overcoming Challenges with Legacy System Integration: Many financial institutions depend on outdated IT infrastructure and systems, which might not integrate seamlessly with AI and ML technologies. Integrating AI/ML solutions with existing systems, data repositories, and workflows is a technical challenge. It requires careful planning and execution to ensure data integrity and system stability.
Conclusion
Quality engineering solutions are digitally transforming wealth management by enhancing testing processes, data quality, predictive capabilities, early anomaly identification, task automation, and refined decision-making. This integration empowers financial institutions to deliver best-of-the-field software solutions, mitigate risks, adhere to regulatory standards, and amplify customer satisfaction.
Bibliography